The Explainability Imperative: Why Businesses Must Prioritize Transparent AI
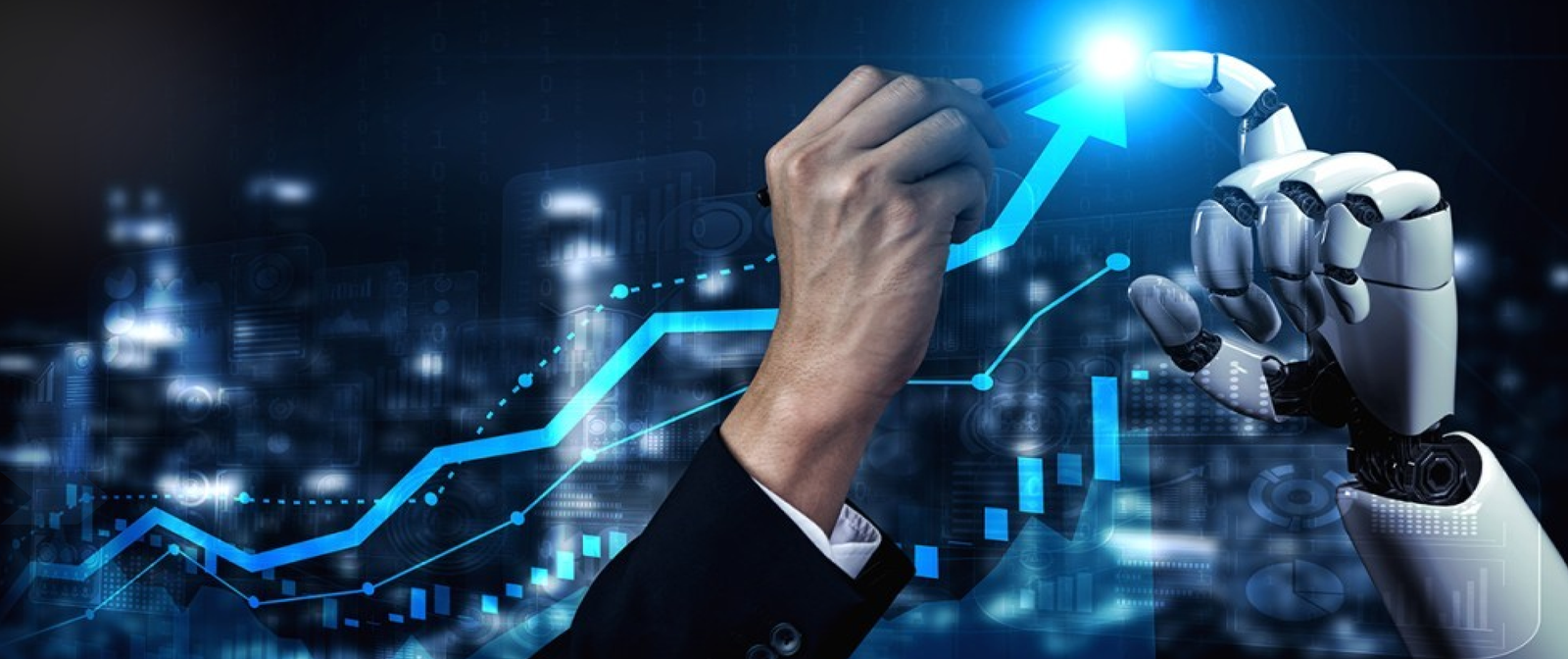
Artificial Intelligence (AI) has transformed from a futuristic concept to a critical driver of business innovation. Today, AI algorithms determine creditworthiness, recommend products, predict market trends, and even diagnose medical conditions. However, as reliance on AI grows, so does the concern over its opacity. Often referred to as “black box models,” many AI systems deliver outcomes without explaining the logic behind their decisions.
The lack of transparency in AI systems poses significant risks. From biased hiring practices to unjust loan rejections, businesses face reputational damage and regulatory repercussions when AI decisions cannot be explained. In an era where trust and accountability are paramount, prioritizing explainable AI (XAI) is not just a technical requirement but a strategic business imperative. This article delves into why businesses must embrace XAI, the challenges involved, and how they can build a roadmap for success.
Introduction: Setting the Stage
The Rise of AI and the Black Box Problem
AI’s ability to process vast amounts of data and derive actionable insights has made it indispensable across industries. However, the trade-off for this advanced capability often lies in reduced interpretability. Complex algorithms like deep learning excel in performance but provide little to no insight into their inner workings. This has led to a phenomenon known as the “black box problem,” where decision-making processes remain hidden from human understanding.
This opacity is not just a technical limitation; it is a business risk. Imagine an insurance company denying coverage based on an AI model’s prediction of risk. If challenged in court or by regulators, the inability to explain the decision could lead to legal penalties and customer attrition. Businesses must recognize that the black box nature of AI is an obstacle to trust, compliance, and effective decision-making.
The argument for explainable AI extends beyond ethical considerations. It is about empowering businesses to build trust, ensure accountability, and maintain compliance in an increasingly AI-driven world. This article outlines the importance of XAI, explores its challenges, and provides actionable strategies for implementation.
The Importance of Explainability in AI
Defining Explainability
Explainability in AI refers to the ability to make an AI system’s decisions comprehensible to humans. It involves not only describing how a decision was reached but also why certain inputs influenced the outcome. This level of transparency is critical for businesses seeking to build stakeholder confidence and avoid the pitfalls of opaque decision-making.
Key Business Impacts
- Building Stakeholder Trust:
Trust is the foundation of successful AI adoption. A McKinsey report highlights that organizations adopting explainable AI experience higher customer satisfaction and stronger adoption rates. Transparent systems allow businesses to demonstrate accountability, enabling customers and partners to place greater confidence in their decisions. - Ensuring Regulatory Compliance:
The legal landscape around AI is becoming increasingly stringent. Regulations like the European Union’s General Data Protection Regulation (GDPR) mandate that individuals have the right to understand how automated decisions are made, particularly when these decisions significantly affect them. Failing to provide such transparency could lead to severe penalties, as seen in cases involving discriminatory algorithms in hiring and lending. - Mitigating Bias and Ethical Risks:
AI systems are only as good as the data they are trained on. When datasets contain biases, AI models can perpetuate and even amplify these issues. For example, a widely reported case involved an AI hiring tool that favored male candidates due to biased training data. Explainable AI enables organizations to identify and address such biases before they cause harm.
Drivers for Explainability
Regulatory Landscape
Governments and regulatory bodies worldwide are recognizing the risks posed by opaque AI systems. In addition to GDPR, the EU’s AI Act classifies certain AI applications as high-risk, requiring rigorous transparency measures. Similarly, the U.S. Federal Trade Commission (FTC) has issued guidance warning companies against deploying AI that cannot be explained. These regulations underscore a growing consensus: businesses must ensure their AI systems are interpretable and accountable.
Customer Expectations
Today’s customers are more informed and discerning about the technologies that impact their lives. A 2023 PwC survey revealed that 78% of consumers prioritize transparency in AI-driven decisions. For example, when a retail platform recommends products, customers are more likely to engage if they understand why certain items were suggested. Failing to meet these expectations can result in lost trust and diminished brand loyalty.
Ethical and Societal Considerations
The ethical implications of AI cannot be ignored. High-profile cases of AI systems perpetuating racial, gender, or socio-economic biases have sparked public outrage and calls for reform. By prioritizing explainability, businesses can align their operations with societal values and demonstrate their commitment to ethical practices. This is particularly critical in sensitive sectors like healthcare, where patient trust is paramount.
Challenges to Achieving Explainability
Technical Complexities
The complexity of modern AI models poses a significant challenge to explainability. Techniques like deep learning involve millions of parameters and intricate layers of computation, making their decision-making processes difficult to interpret. Tools like SHAP (SHapley Additive exPlanations) and LIME (Local Interpretable Model-Agnostic Explanations) are helping address this challenge, but they are not foolproof and require expertise to implement effectively.
Organizational Barriers
Many organizations lack the internal expertise needed to develop explainable AI systems. Data scientists often focus on optimizing model performance, while business leaders may not fully grasp the importance of transparency. Bridging this gap requires a cultural shift that prioritizes cross-functional collaboration and invests in upskilling teams.
Balancing Accuracy and Interpretability
One of the most debated trade-offs in AI development is between accuracy and interpretability. While simpler models like decision trees are easier to explain, they may lack the predictive power of complex algorithms. Businesses must carefully evaluate their priorities, balancing the need for high-performing models with the ethical and operational benefits of transparency.
Strategies for Implementing Explainable AI
Frameworks and Tools
Implementing explainable AI starts with adopting the right tools. Frameworks like LIME and SHAP provide insights into how individual features influence predictions, making it easier to interpret complex models. Additionally, businesses can explore tools like IBM’s AI Explainability 360, which offers a suite of techniques to improve model transparency.
Cross-Functional Collaboration
Explainability is not just a technical challenge—it’s an organizational one. Businesses must foster collaboration between data scientists, compliance officers, and business leaders to ensure AI systems meet technical, ethical, and regulatory standards. This collaborative approach ensures that explainability becomes a shared responsibility across the organization.
Continuous Monitoring
AI systems evolve over time, particularly as they are retrained on new data. Continuous monitoring ensures that explainability remains intact and that any deviations in model behavior are promptly addressed. This is especially critical for industries like finance and healthcare, where the stakes of flawed AI decisions are exceptionally high.
The Role of Human-Centric Design in Explainability
Human-Centric AI and Transparency
Explainable AI aligns perfectly with iauro’s focus on human-centric design. By placing the end user at the center of AI development, businesses can create systems that are not only powerful but also intuitive and trustworthy. For example, a human-centric recommendation system in e-commerce can explain why certain products are suggested, enhancing user engagement and satisfaction.
Empowering End-Users
Transparent AI systems empower end-users by giving them the information they need to make informed decisions. In sectors like healthcare, explainable AI can help patients understand treatment recommendations, fostering trust and collaboration between patients and medical professionals.
Case Study: Healthcare
One notable example of explainable AI in action comes from IBM Watson Health. The platform uses transparent algorithms to assist doctors in diagnosing diseases. By providing clear reasoning for its recommendations, Watson not only supports clinical decision-making but also builds confidence among healthcare providers and patients alike.
The Future of Explainable AI
Emerging Trends
Advancements in interpretable machine learning, such as counterfactual explanations and causal models, promise to make AI even more transparent. These emerging techniques will enable businesses to provide granular insights into their AI systems, further enhancing trust and accountability.
Long-Term Impacts
Explainability will play a defining role in the future of AI adoption. Industries like finance, healthcare, and manufacturing are likely to lead the way, as the risks of opaque AI systems are particularly pronounced in these sectors. Businesses that invest in explainability today will gain a competitive edge and position themselves as leaders in ethical AI.
Conclusion:
The case for explainable AI is clear. As organizations continue to rely on AI for critical decision-making, transparency is no longer a luxury—it’s a necessity. By prioritizing XAI, businesses can build trust, ensure compliance, and operate ethically in an increasingly complex world.
At iauro, we specialize in building human-centric AI systems that prioritize transparency and trust. Contact us to learn how our expertise in explainable AI can help your organization achieve its goals while staying ahead of regulatory and ethical challenges.
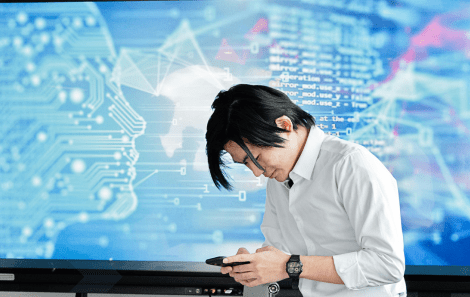
Taking one liner ideas to make impactful business outcomes.
The Explainability Imperative: Why Businesses Must Prioritize Transparent AI
Artificial Intelligence (AI) has transformed from a futuristic concept to a critical driver of business innovation. Today, AI algorithms determine creditworthiness, recommend products, predict market trends, and even diagnose medical conditions. However, as reliance on AI grows, so does the concern over its opacity. Often referred to as “black box models,” many AI systems deliver outcomes without explaining the logic behind their decisions.
The lack of transparency in AI systems poses significant risks. From biased hiring practices to unjust loan rejections, businesses face reputational damage and regulatory repercussions when AI decisions cannot be explained. In an era where trust and accountability are paramount, prioritizing explainable AI (XAI) is not just a technical requirement but a strategic business imperative. This article delves into why businesses must embrace XAI, the challenges involved, and how they can build a roadmap for success.
Introduction: Setting the Stage
The Rise of AI and the Black Box Problem
AI’s ability to process vast amounts of data and derive actionable insights has made it indispensable across industries. However, the trade-off for this advanced capability often lies in reduced interpretability. Complex algorithms like deep learning excel in performance but provide little to no insight into their inner workings. This has led to a phenomenon known as the “black box problem,” where decision-making processes remain hidden from human understanding.
This opacity is not just a technical limitation; it is a business risk. Imagine an insurance company denying coverage based on an AI model’s prediction of risk. If challenged in court or by regulators, the inability to explain the decision could lead to legal penalties and customer attrition. Businesses must recognize that the black box nature of AI is an obstacle to trust, compliance, and effective decision-making.
The argument for explainable AI extends beyond ethical considerations. It is about empowering businesses to build trust, ensure accountability, and maintain compliance in an increasingly AI-driven world. This article outlines the importance of XAI, explores its challenges, and provides actionable strategies for implementation.
The Importance of Explainability in AI
Defining Explainability
Explainability in AI refers to the ability to make an AI system’s decisions comprehensible to humans. It involves not only describing how a decision was reached but also why certain inputs influenced the outcome. This level of transparency is critical for businesses seeking to build stakeholder confidence and avoid the pitfalls of opaque decision-making.
Key Business Impacts
- Building Stakeholder Trust: Trust is the foundation of successful AI adoption. A McKinsey report highlights that organizations adopting explainable AI experience higher customer satisfaction and stronger adoption rates. Transparent systems allow businesses to demonstrate accountability, enabling customers and partners to place greater confidence in their decisions.
- Ensuring Regulatory Compliance: Trust is the foundation of successful AI adoption. A McKinsey report highlights that organizations adopting explainable AI experience higher customer satisfaction and stronger adoption rates. Transparent systems allow businesses to demonstrate accountability, enabling customers and partners to place greater confidence in their decisions.
- Mitigating Bias and Ethical Risks: AI systems are only as good as the data they are trained on. When datasets contain biases, AI models can perpetuate and even amplify these issues. For example, a widely reported case involved an AI hiring tool that favored male candidates due to biased training data. Explainable AI enables organizations to identify and address such biases before they cause harm.
Drivers for Explainability
Regulatory Landscape
Governments and regulatory bodies worldwide are recognizing the risks posed by opaque AI systems. In addition to GDPR, the EU’s AI Act classifies certain AI applications as high-risk, requiring rigorous transparency measures. Similarly, the U.S. Federal Trade Commission (FTC) has issued guidance warning companies against deploying AI that cannot be explained. These regulations underscore a growing consensus: businesses must ensure their AI systems are interpretable and accountable.
Customer Expectations
Today’s customers are more informed and discerning about the technologies that impact their lives. A 2023 PwC survey revealed that 78% of consumers prioritize transparency in AI-driven decisions. For example, when a retail platform recommends products, customers are more likely to engage if they understand why certain items were suggested. Failing to meet these expectations can result in lost trust and diminished brand loyalty.
Ethical and Societal Considerations
The ethical implications of AI cannot be ignored. High-profile cases of AI systems perpetuating racial, gender, or socio-economic biases have sparked public outrage and calls for reform. By prioritizing explainability, businesses can align their operations with societal values and demonstrate their commitment to ethical practices. This is particularly critical in sensitive sectors like healthcare, where patient trust is paramount.
Challenges to Achieving Explainability
Technical Complexities
The complexity of modern AI models poses a significant challenge to explainability. Techniques like deep learning involve millions of parameters and intricate layers of computation, making their decision-making processes difficult to interpret. Tools like SHAP (SHapley Additive exPlanations) and LIME (Local Interpretable Model-Agnostic Explanations) are helping address this challenge, but they are not foolproof and require expertise to implement effectively.
Organizational Barriers
Many organizations lack the internal expertise needed to develop explainable AI systems. Data scientists often focus on optimizing model performance, while business leaders may not fully grasp the importance of transparency. Bridging this gap requires a cultural shift that prioritizes cross-functional collaboration and invests in upskilling teams.
Balancing Accuracy and Interpretability
One of the most debated trade-offs in AI development is between accuracy and interpretability. While simpler models like decision trees are easier to explain, they may lack the predictive power of complex algorithms. Businesses must carefully evaluate their priorities, balancing the need for high-performing models with the ethical and operational benefits of transparency.
Strategies for Implementing Explainable AI
Frameworks and Tools
Implementing explainable AI starts with adopting the right tools. Frameworks like LIME and SHAP provide insights into how individual features influence predictions, making it easier to interpret complex models. Additionally, businesses can explore tools like IBM’s AI Explainability 360, which offers a suite of techniques to improve model transparency.
Cross-Functional Collaboration
Explainability is not just a technical challenge—it’s an organizational one. Businesses must foster collaboration between data scientists, compliance officers, and business leaders to ensure AI systems meet technical, ethical, and regulatory standards. This collaborative approach ensures that explainability becomes a shared responsibility across the organization.
Continuous Monitoring
AI systems evolve over time, particularly as they are retrained on new data. Continuous monitoring ensures that explainability remains intact and that any deviations in model behavior are promptly addressed. This is especially critical for industries like finance and healthcare, where the stakes of flawed AI decisions are exceptionally high.
The Role of Human-Centric Design in Explainability
Human-Centric AI and Transparency
Explainable AI aligns perfectly with iauro’s focus on human-centric design. By placing the end user at the center of AI development, businesses can create systems that are not only powerful but also intuitive and trustworthy. For example, a human-centric recommendation system in e-commerce can explain why certain products are suggested, enhancing user engagement and satisfaction.
Empowering End-Users
Transparent AI systems empower end-users by giving them the information they need to make informed decisions. In sectors like healthcare, explainable AI can help patients understand treatment recommendations, fostering trust and collaboration between patients and medical professionals.
Case Study: Healthcare
One notable example of explainable AI in action comes from IBM Watson Health. The platform uses transparent algorithms to assist doctors in diagnosing diseases. By providing clear reasoning for its recommendations, Watson not only supports clinical decision-making but also builds confidence among healthcare providers and patients alike.
The Future of Explainable AI
Emerging Trends
Advancements in interpretable machine learning, such as counterfactual explanations and causal models, promise to make AI even more transparent. These emerging techniques will enable businesses to provide granular insights into their AI systems, further enhancing trust and accountability.
Long-Term Impacts
Explainability will play a defining role in the future of AI adoption. Industries like finance, healthcare, and manufacturing are likely to lead the way, as the risks of opaque AI systems are particularly pronounced in these sectors. Businesses that invest in explainability today will gain a competitive edge and position themselves as leaders in ethical AI.
Conclusion:
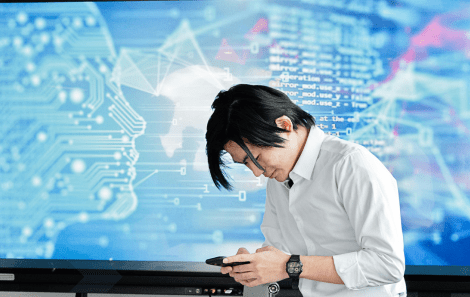
The case for explainable AI is clear. As organizations continue to rely on AI for critical decision-making, transparency is no longer a luxury—it’s a necessity. By prioritizing XAI, businesses can build trust, ensure compliance, and operate ethically in an increasingly complex world.
At iauro, we specialize in building human-centric AI systems that prioritize transparency and trust. Contact us to learn how our expertise in explainable AI can help your organization achieve its goals while staying ahead of regulatory and ethical challenges.
Taking one liner ideas to make impactful business outcomes.