Traditional AI vs Gen AI : Decoding the AI Landscape
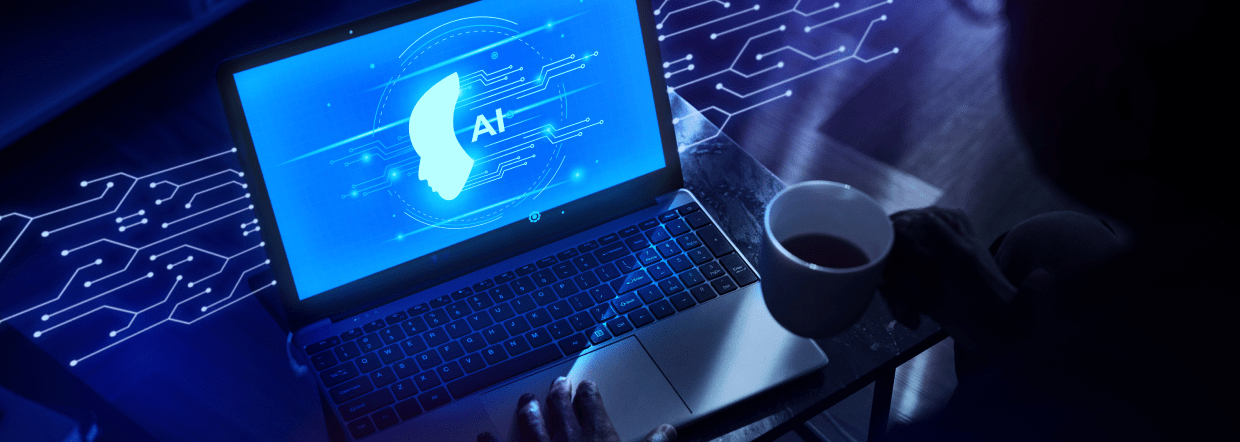
Introduction:
Key Differences Between Traditional AI vs Gen AI
Conventional AI primarily utilizes models that differentiate between classes based on explicit rules.
Generative AI on the other hand relies on models that create fresh instances and content by understanding the underlying patterns present in the data.
Key Characteristics and Principles
Traditional AI is known for its rule-based nature, where explicit rules and algorithms govern decision making. Its main features include transparency and predictability making it suitable for tasks with defined rules.
Real World Applications
Traditional AI excels in scenarios, where structured decision making is crucial. Industries such as automation and expert systems in medicine heavily rely on Traditional AI due to its stability and consistency.
Meaning of Generative AI
Generative AI takes a creative approach. It involves training models to generate content whether it's language, images or complete pieces of art. Prominent techniques like Generative Adversarial Networks (GANs) and language models like Generative Pre trained Transformers (GPT) fall under the umbrella of Generative AI.
Real World Applications
Generative AI demonstrates its proficiency in fields such as art, music and content generation.
It plays a role in producing results making it a driving force in the advancement of deepfakes and other cutting-edge applications.
Advantages and Disadvantages
- Transparency; The clear definition of rules ensures a decision-making process.
- Stability; Traditional AI systems are generally stable and predictable which makes them suitable for applications, where consistency essential.
- Limited Adaptability; Traditional AI faces difficulties in environments where rules may require updates.
- Dependence on Human Expertise; Success heavily relies on expressing expertise.
- Creativity; The ability of AI to create content is invaluable in creative domains like art and music
- Adaptability; Generative models can adjust to datasets generating outputs, for scenarios.
- Lack of Control; The creative outputs may not always align with requirements posing challenges in managing the process.
- 2. Ethical Concerns; The ethical implications surrounding the use of models, such, as deepfakes are centred around issues of privacy and the spread of misinformation
Applications and Future Prospects
In summary, both Traditional AI vs Gen AI approaches have their strengths and limitations. Understanding these differences is essential for leveraging the appropriate AI technology in various applications. The future of AI may involve an integration of these approaches combining the decision-making capabilities of traditional AI with the creative potential of generative AI to tackle complex problems, across various domains.
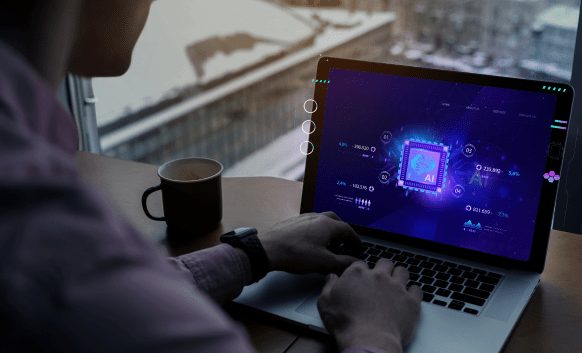
Conventional AI primarily utilizes models that differentiate between classes based on explicit rules.
Generative AI on the other hand relies on models that create fresh instances and content by understanding the underlying patterns present in the data.
Traditional AI
Key Characteristics and Principles
Traditional AI is known for its rule-based nature, where explicit rules and algorithms govern decision making. Its main features include transparency and predictability making it suitable for tasks with defined rules.
Real World Applications
Traditional AI excels in scenarios, where structured decision making is crucial. Industries such as automation and expert systems in medicine heavily rely on Traditional AI due to its stability and consistency.
Meaning of Generative AI
Generative AI takes a creative approach. It involves training models to generate content whether it's language, images or complete pieces of art. Prominent techniques like Generative Adversarial Networks (GANs) and language models like Generative Pre trained Transformers (GPT) fall under the umbrella of Generative AI.
Real World Applications
Generative AI takes a creative approach. It involves training models to generate content whether it's language, images or complete pieces of art. Prominent techniques like Generative Adversarial Networks (GANs) and language models like Generative Pre trained Transformers (GPT) fall under the umbrella of Generative AI.
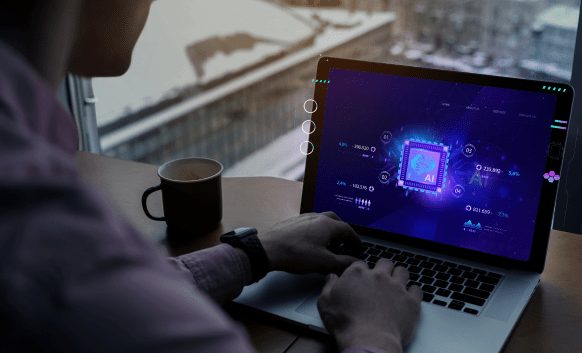
In summary, both Traditional AI vs Gen AI approaches have their strengths and limitations. Understanding these differences is essential for leveraging the appropriate AI technology in various applications. The future of AI may involve an integration of these approaches combining the decision-making capabilities of traditional AI with the creative potential of generative AI to tackle complex problems, across various domains.