Data Dilemma in Generative AI Quality vs Quantity
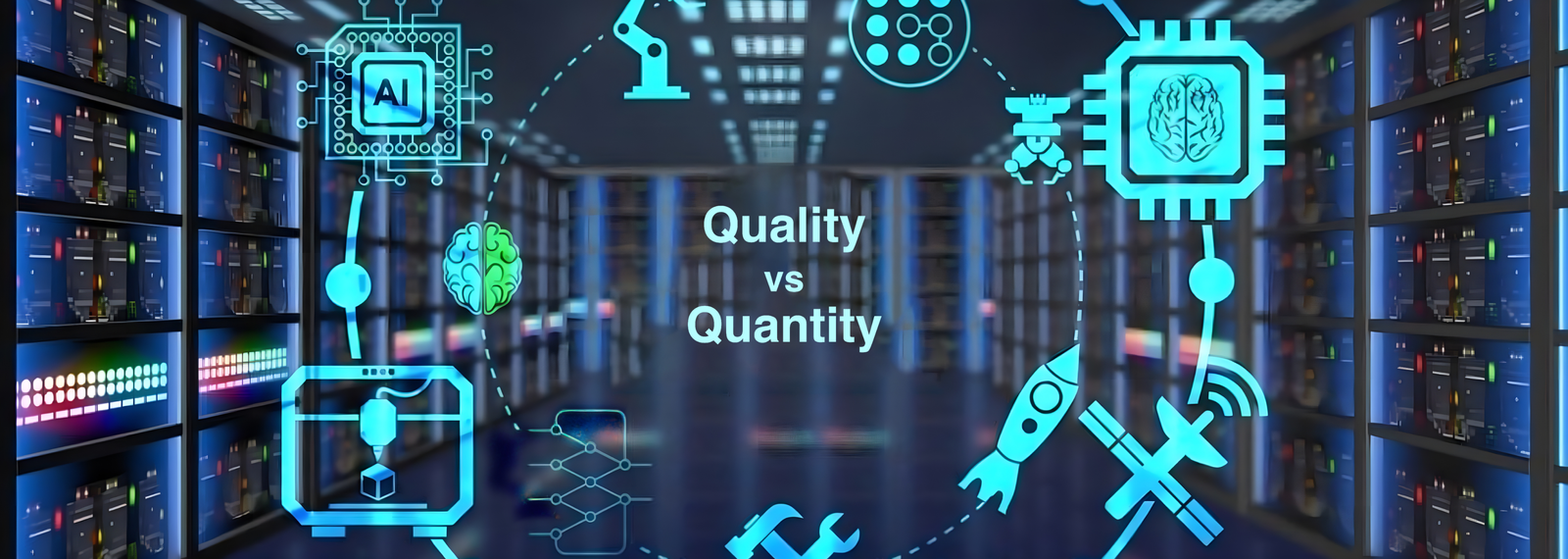
Consideration of Data in GenAI
Quantity vs Diversity
Domain- Specific Considerations
Different domains pose unique challenges and considerations regarding data in GenAI. For instance, in healthcare, ensuring patient privacy and compliance with regulatory frameworks like HIPAA is paramount. Similarly, in finance, data security and confidentiality are non-negotiable. Understanding the intricacies of each domain and tailoring data collection and preprocessing strategies accordingly is essential to the successful deployment of GenAI solutions.
Data Readiness Assessment
Before embarking on GenAI projects, conducting a comprehensive data readiness assessment is indispensable. This assessment involves evaluating the availability, quality, and suitability of existing data sets for training and validation purposes. Additionally, identifying potential gaps or biases in the data and devising strategies to address them is critical. By conducting a thorough data readiness assessment, organizations can mitigate risks and set realistic expectations for GenAI projects.
Conclusion
The data dilemma in Generative AI presents both challenges and opportunities for organizations seeking to leverage AI-driven solutions effectively. By carefully considering aspects such as data identification, quantity, diversity, quality, domain-specific considerations, and data readiness assessment, organizations can navigate this dilemma adeptly. Ultimately, prioritizing the integrity and suitability of data sets lays the foundation for the development of robust and ethically sound GenAI applications that drive innovation and value creation across diverse domains.
In the world of GenAI, the interplay between data quality and quantity is a pivotal factor influencing the efficacy of models and the outcomes they generate. As organizations delve into harnessing the power of GenAI, navigating the delicate balance between these two aspects becomes imperative. In this blog, we will explore the considerations surrounding data in GenAI, focusing on data identification and collection, the debate between quantity and diversity, the importance of data quality, domain-specific considerations, and the significance of data readiness assessment.
Data serves as the lifeblood of Generative AI systems, shaping their capabilities and performance. The first step in leveraging GenAI effectively lies in the meticulous identification and collection of relevant data sets. This process involves sourcing data from diverse and reputable sources, ensuring its relevance to the intended application, and adhering to ethical guidelines governing data usage.
The onboarding experience sets the tone for user engagement and retention. In a UX audit, designers assess the effectiveness of the onboarding process in guiding users through the product’s features and functionalities. They evaluate the clarity of instructions, the simplicity of tasks, and the provision of relevant guidance to help users get started quickly and confidently.
The quality of data utilized for training GenAI models profoundly influences their performance and outcomes. High-quality data is characterized by accuracy, completeness, and relevance to the task at hand. Moreover, mitigating biases inherent in the data is paramount to prevent perpetuating unfair or discriminatory outcomes. Rigorous preprocessing techniques, such as data anonymization and bias mitigation algorithms, play a crucial role in enhancing the quality and fairness of GenAI models.
Different domains pose unique challenges and considerations regarding data in GenAI. For instance, in healthcare, ensuring patient privacy and compliance with regulatory frameworks like HIPAA is paramount. Similarly, in finance, data security and confidentiality are non-negotiable. Understanding the intricacies of each domain and tailoring data collection and preprocessing strategies accordingly is essential to the successful deployment of GenAI solutions.
Sustaining user engagement over time is essential for the success of any digital product. In a UX audit, designers analyze factors that contribute to long-term user engagement, such as the availability of fresh content, interactive features, personalized recommendations, and gamification elements. By fostering ongoing engagement, organizations can cultivate loyal users who return to the product regularly and advocate for it within their networks.
The data dilemma in Generative AI presents both challenges and opportunities for organizations seeking to leverage AI-driven solutions effectively. By carefully considering aspects such as data identification, quantity, diversity, quality, domain-specific considerations, and data readiness assessment, organizations can navigate this dilemma adeptly. Ultimately, prioritizing the integrity and suitability of data sets lays the foundation for the development of robust and ethically sound GenAI applications that drive innovation and value creation across diverse domains.